The insurance industry is undergoing a significant transformation driven by the rapid advancements in artificial intelligence (AI). AI’s ability to analyze vast datasets, identify patterns, and automate processes is revolutionizing how insurers assess risk, detect fraud, and interact with customers. This exploration delves into the multifaceted impact of AI across various aspects of insurance, highlighting both its transformative potential and the challenges it presents.
From AI-powered risk assessment that improves accuracy and speed to AI-driven chatbots enhancing customer service, the applications are wide-ranging. We will examine how AI is personalizing insurance products, streamlining claims processing, and ultimately reshaping the future of the industry. The ethical considerations and potential biases associated with AI implementation will also be addressed, ensuring a balanced perspective on this rapidly evolving landscape.
AI-Driven Risk Assessment in Insurance
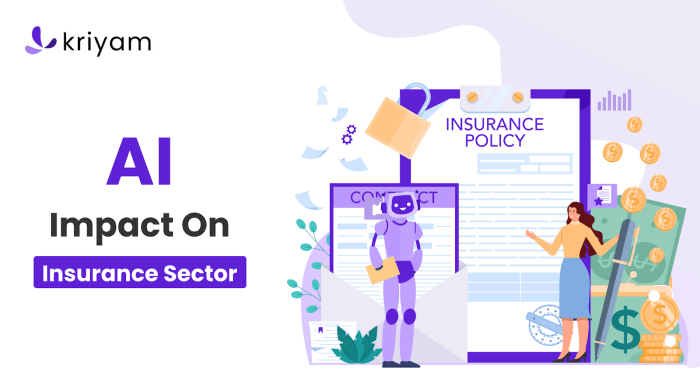
AI is revolutionizing risk assessment in the insurance industry, offering unprecedented accuracy and efficiency. Traditional methods often rely on limited data points and human judgment, leading to potential biases and inaccuracies. AI, however, leverages vast datasets and sophisticated algorithms to analyze complex patterns and predict risks with greater precision. This leads to more accurate pricing, improved underwriting decisions, and ultimately, a more sustainable and equitable insurance market.
AI Algorithms for Enhanced Risk Assessment
AI algorithms excel at risk assessment by analyzing far more data points than human underwriters can practically manage. Machine learning models, for example, can identify subtle correlations between seemingly disparate data sources to create a more holistic risk profile. This includes factors often overlooked in traditional assessments, such as social media activity, geolocation data, and even sensor data from connected devices. Deep learning models, a subset of machine learning, can further enhance this process by identifying complex, non-linear relationships within the data. These advanced algorithms are particularly effective in detecting fraud and predicting high-risk behaviors.
AI vs. Human-Driven Risk Assessment
AI-powered risk assessment offers several advantages over human-driven approaches. It significantly increases speed and efficiency, processing vast amounts of data in a fraction of the time it would take a human. Furthermore, AI eliminates human biases, leading to fairer and more objective assessments. However, AI models are only as good as the data they are trained on, and potential biases embedded in the data can lead to unfair outcomes. Human oversight remains crucial to ensure the ethical and responsible use of AI in risk assessment. Human underwriters still provide valuable context and judgment in cases requiring nuanced interpretation or where unusual circumstances necessitate a more holistic view.
Data Used in AI-Driven Risk Assessment and Data Privacy
AI-driven risk assessment utilizes a wide range of data, including demographic information, credit scores, driving records, claims history, telematics data (from connected car devices), and even social media activity (with appropriate consent). Data privacy is paramount, and stringent measures are employed to protect sensitive information. This includes anonymization techniques, data encryption, and adherence to strict regulatory frameworks like GDPR and CCPA. Data governance practices, such as implementing robust access controls and regular audits, are also essential to maintaining data integrity and ensuring compliance.
Hypothetical Scenario: AI-Enhanced Underwriting
Imagine a scenario where an individual applies for car insurance. Traditionally, underwriting would involve reviewing a limited set of data points, such as driving history and credit score. With AI, the process is enhanced. The AI system analyzes telematics data from the applicant’s vehicle, revealing consistent safe driving behavior, even if their driving record shows a minor infraction years ago. Additionally, the AI system cross-references this data with other sources, such as social media activity (with consent) to gain a comprehensive picture of their lifestyle and risk profile. The result is a more accurate risk assessment, leading to a potentially lower premium for the applicant based on their demonstrably safe driving habits.
Cost-Effectiveness of AI-Driven vs. Traditional Risk Assessment
Feature | AI-Driven Risk Assessment | Traditional Risk Assessment |
---|---|---|
Initial Investment | High (software, data acquisition, infrastructure) | Low (primarily human salaries) |
Operational Costs | Lower (automation reduces manual labor) | Higher (salaries, administrative overhead) |
Accuracy | Higher (more data points, reduced bias) | Lower (reliance on limited data and human judgment) |
Speed | Significantly faster | Slower (manual data processing) |
AI in Fraud Detection and Prevention
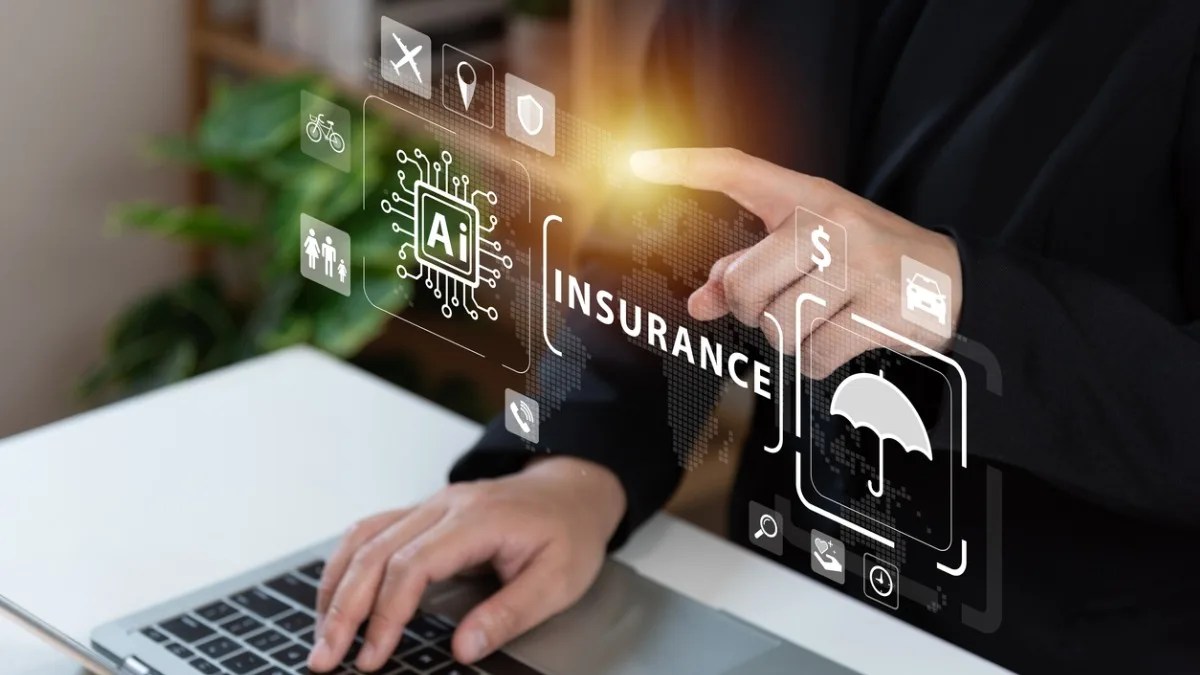
The insurance industry faces significant financial losses due to fraudulent activities. AI offers a powerful toolset to combat this, enabling insurers to detect and prevent fraud more effectively than traditional methods. By analyzing vast datasets and identifying complex patterns, AI algorithms can significantly improve the accuracy and efficiency of fraud detection.
AI algorithms can identify patterns indicative of insurance fraud through sophisticated analysis of various data points. These algorithms are trained on massive datasets containing historical claims data, policyholder information, and external data sources. They look for anomalies and deviations from established norms, flagging suspicious patterns that might indicate fraudulent behavior. For example, an unusually high number of claims from a specific geographic area, or claims with suspiciously similar details across multiple policies, might trigger an alert. The algorithms can also analyze unstructured data like claim narratives and medical reports, identifying inconsistencies or red flags that might be missed by human reviewers.
AI-Powered Tools for Fraud Detection
Several AI-powered tools are currently employed in detecting fraudulent claims. These tools often leverage machine learning techniques such as anomaly detection, predictive modeling, and natural language processing (NLP). One common approach involves building predictive models that assign a risk score to each claim based on various factors. Claims with high risk scores are then flagged for further investigation by human fraud investigators. Another tool utilizes NLP to analyze the textual descriptions within claims, identifying inconsistencies or discrepancies that might indicate fraud. For example, an NLP algorithm might detect inconsistencies between a claimant’s description of an accident and witness statements or medical reports. Furthermore, network analysis techniques can identify clusters of potentially fraudulent claims linked through common characteristics or individuals. This allows insurers to identify organized fraud rings.
Ethical Considerations of AI in Fraud Detection
The use of AI in fraud detection raises important ethical considerations. One key concern is the potential for bias in algorithms. If the training data reflects existing biases, the AI system may unfairly target certain demographic groups. This necessitates careful selection and preprocessing of training data to mitigate bias. Another concern is data privacy. AI systems often require access to sensitive personal information, raising concerns about data security and potential misuse. Robust data protection measures are crucial to ensure compliance with privacy regulations and maintain public trust. Finally, the potential for false positives – wrongly accusing legitimate claimants of fraud – needs careful consideration. Striking a balance between effective fraud detection and protecting the rights of honest claimants is a crucial ethical challenge.
Reducing Financial Losses Through AI
AI can significantly reduce financial losses due to fraudulent activities. By proactively identifying and preventing fraudulent claims, insurers can save substantial sums of money. The improved accuracy and efficiency of AI-driven fraud detection systems lead to quicker identification of fraudulent claims, reducing the time and resources spent on investigations. Moreover, AI can help prevent fraudulent claims altogether by identifying high-risk applicants or policies before they are even issued. This proactive approach minimizes the opportunity for fraud. The cumulative effect of these improvements translates to significant cost savings for insurers. For example, a study by a leading insurance company showed a 20% reduction in fraudulent claims after implementing an AI-powered fraud detection system.
Integrating AI-Driven Fraud Detection Systems
Integrating AI-driven fraud detection systems into existing insurance workflows requires a phased approach. Initially, insurers may start by piloting the AI system on a subset of claims to evaluate its performance and identify areas for improvement. This allows for iterative refinement of the system based on real-world data and feedback. As the system’s accuracy and reliability improve, it can be gradually integrated into the broader claims processing workflow. This may involve integrating the AI system with existing claims management platforms, training staff on how to interpret the AI’s outputs, and establishing clear protocols for handling flagged claims. A successful integration requires careful planning, collaboration between IT and claims departments, and a commitment to ongoing monitoring and improvement of the system.
AI-Powered Customer Service and Chatbots
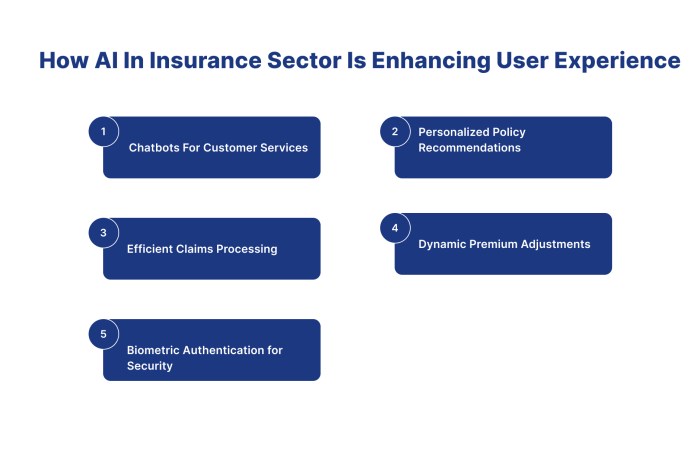
The insurance industry, traditionally reliant on human interaction, is undergoing a significant transformation with the integration of AI-powered customer service and chatbots. These intelligent systems offer the potential to streamline operations, enhance customer experience, and improve efficiency across various aspects of insurance services. This section will explore the role of AI chatbots in revolutionizing customer service within the insurance sector.
AI chatbots are rapidly becoming indispensable tools for improving customer service in the insurance industry. They offer 24/7 availability, instant responses, and personalized interactions, leading to increased customer satisfaction and reduced operational costs. By automating routine tasks and handling a large volume of inquiries simultaneously, they free up human agents to focus on more complex and nuanced issues requiring specialized expertise.
AI Chatbot Handling of Common Customer Inquiries
AI chatbots in insurance can effectively address a wide range of common customer inquiries. For example, they can provide policy information (coverage details, premium amounts, renewal dates), answer frequently asked questions about claims procedures (reporting a claim, required documentation, claim status updates), and offer guidance on policy changes or add-ons. They can also assist with simple tasks like updating contact information or providing general information about the insurance company’s products and services. A sophisticated chatbot might even be able to process simple policy changes or initiate claims based on pre-defined criteria.
Comparison of AI Chatbots and Human Agents in Addressing Customer Needs
While AI chatbots excel at handling routine inquiries efficiently and at scale, human agents remain crucial for addressing complex or emotionally charged situations. Chatbots are generally more cost-effective for high-volume, simple requests, but lack the empathy and nuanced understanding that a human agent can provide. For example, a chatbot might struggle to comfort a customer who has experienced a significant loss, whereas a human agent can offer personalized support and understanding. The ideal scenario often involves a collaborative approach, where chatbots handle initial inquiries and escalate complex issues to human agents.
Conversational Flow for an AI Chatbot Handling Policy Inquiries
A well-designed chatbot for policy inquiries should follow a structured conversational flow. For instance:
1. Greeting and Identification: The chatbot greets the customer and asks for their policy number or other identifying information.
2. Inquiry Clarification: The chatbot asks clarifying questions to understand the customer’s specific needs. For example, “What information about your policy are you looking for?”
3. Information Retrieval and Delivery: The chatbot retrieves the relevant information from the database and presents it to the customer in a clear and concise manner.
4. Additional Assistance: The chatbot offers additional assistance or directs the customer to relevant resources.
5. Closing: The chatbot thanks the customer for their inquiry and provides options for further contact if needed.
This flow ensures a smooth and efficient interaction, minimizing customer frustration and maximizing the chatbot’s effectiveness.
Benefits and Challenges of Implementing AI-Powered Customer Service in Insurance
Implementing AI-powered customer service in insurance offers several significant benefits, including reduced operational costs, improved customer satisfaction through 24/7 availability and faster response times, increased efficiency by automating routine tasks, and the ability to handle a larger volume of inquiries simultaneously. However, challenges remain. These include the need for substantial upfront investment in technology and training, the potential for inaccurate or unhelpful responses from chatbots if not properly trained, and the need to ensure data privacy and security. Moreover, the complete replacement of human interaction could lead to a perceived lack of empathy and personalized service, highlighting the need for a balanced approach integrating both AI and human agents.
AI and Personalized Insurance Products
The application of artificial intelligence (AI) is revolutionizing the insurance industry, moving beyond simple automation to create highly personalized products and services. By leveraging vast datasets and sophisticated algorithms, insurers can now tailor policies to individual customer needs with unprecedented accuracy, leading to more competitive offerings and improved customer satisfaction. This personalization extends to pricing, coverage options, and even the overall customer experience.
AI facilitates the development of personalized insurance products by analyzing individual customer data to assess risk more precisely than traditional methods. This data can include demographics, lifestyle choices, driving habits (for auto insurance), health records (for health insurance), and even social media activity, all contributing to a more comprehensive risk profile. Through machine learning, AI algorithms identify patterns and correlations within this data, enabling the creation of insurance policies that accurately reflect the individual’s risk level and, consequently, their premium.
Personalized Insurance Product Examples
AI-driven personalization allows for the creation of insurance products previously unimaginable. For example, usage-based insurance (UBI) for automobiles uses telematics to track driving behavior, rewarding safer drivers with lower premiums. Similarly, in health insurance, AI can analyze individual health data to identify potential risks and offer personalized wellness programs alongside tailored coverage plans. Another example is the emergence of micro-insurance products, offering targeted coverage for specific needs or short durations, made possible by AI’s ability to quickly assess and manage risk for these smaller, more granular policies. These products cater to specific segments of the population who might have been underserved by traditional insurance models.
Ethical Implications of AI-Driven Personalization
The use of AI in insurance pricing and coverage raises important ethical considerations. The primary concern is the potential for algorithmic bias, where AI models may inadvertently discriminate against certain groups based on pre-existing societal biases present in the training data. For instance, if historical data reflects discriminatory lending practices, an AI model trained on this data might perpetuate these biases in insurance pricing, unfairly penalizing certain demographics. Transparency and explainability of AI algorithms are crucial to address this concern, ensuring that decisions are fair and justifiable. Furthermore, the potential for data privacy violations needs careful consideration and robust data protection measures must be implemented. Insurers must be transparent about the data they collect and how it’s used to ensure customer trust.
Potential Biases in AI-Driven Personalization and Mitigation Strategies
AI models are only as good as the data they are trained on. If the data reflects existing societal biases, the AI model will likely perpetuate and even amplify these biases. For example, an AI model trained on data showing a higher accident rate for a specific demographic might unfairly increase premiums for individuals within that group, regardless of their individual driving record. Mitigation strategies include: (1) Ensuring diverse and representative datasets are used for training AI models; (2) Implementing rigorous testing and validation procedures to identify and address potential biases; (3) Employing techniques like fairness-aware machine learning to actively mitigate bias during model development; and (4) establishing independent audits to review AI algorithms for fairness and transparency. These steps are vital to ensuring equitable access to insurance and preventing discriminatory outcomes.
Hypothetical AI-Powered Insurance Product: “SmartHome Protect”
SmartHome Protect is a home insurance product powered by AI that dynamically adjusts premiums based on real-time risk assessment. Using smart home devices like security systems, smoke detectors, and smart locks, the policy continuously monitors the home’s security and safety. If the homeowner implements proactive safety measures, such as installing a monitored security system or upgrading their smoke detectors, their premium is reduced. Conversely, if the system detects risky behaviors or potential vulnerabilities, the policy might temporarily increase the premium as an incentive to address the identified issues. This personalized approach creates a dynamic insurance experience that rewards proactive safety measures and incentivizes responsible homeownership, ultimately leading to lower premiums and a safer living environment for the policyholder. The system also includes a personalized risk assessment report, providing the homeowner with insights and recommendations for improving their home’s security and safety.
AI in Claims Processing and Management
The insurance industry is undergoing a significant transformation driven by the integration of artificial intelligence (AI). AI’s impact is particularly profound in claims processing and management, offering the potential to dramatically improve efficiency, reduce costs, and enhance customer satisfaction. This section explores how AI is revolutionizing this crucial aspect of insurance operations.
AI can automate and streamline the claims processing workflow through various techniques. By leveraging machine learning algorithms, AI systems can analyze vast amounts of data – from policy details and claim forms to medical records and repair estimates – to identify patterns, predict potential fraud, and automate routine tasks. This automation reduces manual intervention, minimizing human error and accelerating the overall claims process.
AI-Powered Tools in Claims Management
Several AI-powered tools are currently employed in claims management. Optical Character Recognition (OCR) software, for example, automatically extracts data from submitted documents, eliminating manual data entry. Natural Language Processing (NLP) tools analyze claim narratives to identify key information and assess the validity of claims. Predictive analytics models forecast claim costs and identify high-risk claims, enabling proactive risk management. Robotic Process Automation (RPA) automates repetitive tasks like data verification and document routing, freeing up human agents to focus on more complex cases. Furthermore, AI-powered chatbots provide immediate support to claimants, answering frequently asked questions and guiding them through the claims process.
Efficiency Comparison: AI vs. Traditional Claims Processing
Traditional claims processing relies heavily on manual review and processing, which is time-consuming, prone to errors, and often leads to processing bottlenecks. AI-driven claims processing, on the other hand, significantly improves efficiency by automating many stages of the process. For example, a study by a leading insurance technology firm showed a 30% reduction in processing time and a 15% reduction in operational costs for insurers who implemented AI-powered claims management systems. This translates to faster claim settlements, increased customer satisfaction, and improved operational efficiency.
AI’s Impact on Processing Time and Customer Satisfaction
AI significantly reduces processing times by automating data extraction, validation, and routing. This speedier process minimizes delays and frustrations for claimants. Furthermore, AI-powered chatbots offer immediate support and personalized communication, addressing claimant queries promptly and efficiently. This proactive and responsive approach significantly enhances customer satisfaction. By providing clear updates and consistent communication throughout the claims process, insurers can build trust and loyalty. A real-world example is seen with several major insurance companies reporting significant increases in customer satisfaction scores after implementing AI-driven claims management systems.
Step-by-Step AI-Enhanced Claims Process
- Claim Reporting: The claimant submits a claim through various channels (online portal, mobile app, phone). AI-powered chatbots guide claimants through the process and collect necessary information.
- Initial Assessment: AI systems use OCR and NLP to automatically extract and analyze information from the claim documents. This includes identifying the type of claim, assessing its validity, and flagging any potential red flags.
- Data Verification: AI automates data verification processes, comparing claim information with policy details and other relevant databases. This helps to identify inconsistencies and potential fraud.
- Investigation and Evaluation: For complex claims, AI provides insights to guide human investigators, prioritizing cases based on risk and potential costs.
- Settlement and Payment: Based on the assessment and investigation, AI helps determine the appropriate settlement amount and automates the payment process.
- Post-Settlement Analysis: AI analyzes the entire claims process to identify areas for improvement and refine the system’s efficiency and accuracy.
The Future of AI in Insurance
The integration of artificial intelligence (AI) is rapidly transforming the insurance industry, promising a future characterized by enhanced efficiency, personalized services, and reduced risk. While significant progress has already been made in areas like fraud detection and claims processing, the next 5-10 years will witness even more profound changes driven by advancements in AI capabilities and increased data availability. This section explores the potential future applications, impacts, challenges, and necessary steps for successful AI adoption within the insurance sector.
Potential Future Applications of AI in Insurance
AI’s potential extends far beyond its current applications. We can anticipate advancements in areas such as predictive modeling for more accurate risk assessment, incorporating real-time data from connected devices (IoT) for personalized premiums and immediate claims processing, and the development of sophisticated AI-powered advisors capable of providing tailored financial planning alongside insurance recommendations. For instance, imagine AI systems analyzing an individual’s driving habits in real-time through a connected car app, instantly adjusting their car insurance premiums based on their driving performance. This level of dynamic pricing and personalized risk assessment is only possible through the sophisticated analytical capabilities of AI. Another example is the use of AI in catastrophe modeling, improving prediction accuracy and allowing for more precise risk management in areas prone to natural disasters.
Impact of AI on the Insurance Industry in the Next 5-10 Years
Over the next 5-10 years, AI is predicted to significantly streamline insurance operations, leading to cost reductions and improved efficiency. Automated processes will reduce manual workloads, freeing up human resources for more complex tasks. We can expect to see a rise in hyper-personalized insurance products, tailored to individual needs and risk profiles, leading to increased customer satisfaction and loyalty. Companies like Lemonade have already demonstrated the power of AI in creating streamlined, customer-centric insurance experiences. However, the impact will also involve significant workforce shifts, requiring retraining and upskilling of employees to adapt to the changing landscape. The industry will likely see a decline in roles focused on repetitive manual tasks, while demand for AI specialists and data scientists will increase dramatically.
Challenges and Opportunities Associated with Widespread AI Adoption in Insurance
The widespread adoption of AI in insurance presents both significant opportunities and considerable challenges. Data privacy and security concerns are paramount. Ensuring the ethical use of AI algorithms and mitigating biases are crucial to maintaining trust and avoiding discriminatory practices. The need for robust regulatory frameworks to govern the use of AI in insurance is also a key challenge. On the opportunity side, AI can unlock significant cost savings, improve operational efficiency, and enhance customer experiences, ultimately leading to greater profitability and competitiveness for insurance companies that successfully embrace this technology. The ability to leverage large datasets to develop more accurate risk models and offer personalized products will be a key differentiator.
Visual Representation of the Future Landscape of AI in Insurance
Imagine a dynamic, interconnected network. At the center is a powerful AI engine, processing vast amounts of data from various sources: telematics data from connected devices, social media analytics, weather patterns, and traditional insurance data. From this central engine, numerous interconnected nodes extend, representing different aspects of the insurance business: automated claims processing, personalized product offerings, fraud detection, risk assessment, and customer service chatbots. These nodes are constantly interacting and exchanging information, creating a seamless and efficient ecosystem. The entire network is surrounded by a layer representing regulatory compliance and data security measures, emphasizing the importance of ethical and responsible AI implementation.
Necessary Steps for Successful AI Integration in Insurance
Successful AI integration requires a multi-faceted approach. Firstly, insurance companies need to invest in robust data infrastructure and analytical capabilities. Secondly, a skilled workforce proficient in AI and data science is essential. Thirdly, a clear strategy outlining the specific business goals AI is intended to achieve is crucial. Finally, a strong emphasis on data privacy, security, and ethical AI practices is non-negotiable. This involves implementing rigorous data governance policies and regularly auditing AI algorithms to ensure fairness and transparency. Companies must also prioritize employee training and development to equip their workforce with the necessary skills to work alongside and manage AI systems effectively.
Epilogue
In conclusion, the integration of AI in the insurance sector presents a paradigm shift, promising enhanced efficiency, accuracy, and customer experience. While challenges remain, particularly concerning data privacy and ethical considerations, the potential benefits are undeniable. The future of insurance is inextricably linked to AI, and embracing its potential responsibly will be crucial for insurers to thrive in the years to come. The ongoing evolution of AI technology promises further innovation and disruption, creating both exciting opportunities and demanding careful navigation of its implications.
FAQ Corner
What are the biggest challenges in implementing AI in insurance?
Significant challenges include data privacy concerns, the need for robust data infrastructure, ensuring algorithmic fairness and mitigating bias, and the high initial investment costs.
How does AI improve customer satisfaction in insurance?
AI enhances customer satisfaction through faster claims processing, 24/7 chatbot support for immediate query resolution, and personalized product offerings tailored to individual needs.
Will AI replace human insurance agents?
While AI automates many tasks, it’s unlikely to entirely replace human agents. The human element remains crucial for complex cases, relationship building, and providing empathetic customer service.
What are the regulatory implications of using AI in insurance?
Regulations are evolving to address data privacy, algorithmic transparency, and the responsible use of AI in decision-making processes. Insurers need to stay abreast of these changes to ensure compliance.